Note
Go to the end to download the full example code
Construct a model RDM#
This example shows how to create RDMs from arbitrary data. A common use case for this is to construct a “model” RDM to RSA against the brain data. In this example, we will create a RDM based on the length of the words shown during an EEG experiment.
# Import required packages
import mne
import mne_rsa
MNE-Python contains a build-in data loader for the kiloword dataset, which is used
here as an example dataset. Since we only need the words shown during the experiment,
which are in the metadata, we can pass preload=False
to prevent MNE-Python from
loading the EEG data, which is a nice speed gain.
data_path = mne.datasets.kiloword.data_path(verbose=True)
epochs = mne.read_epochs(data_path / "kword_metadata-epo.fif", preload=False)
# Show the metadata of 10 random epochs
print(epochs.metadata.sample(10))
Reading /l/vanvlm1/mne_data/MNE-kiloword-data/kword_metadata-epo.fif ...
Isotrak not found
Found the data of interest:
t = -100.00 ... 920.00 ms
0 CTF compensation matrices available
Adding metadata with 8 columns
960 matching events found
No baseline correction applied
0 projection items activated
WORD Concreteness WordFrequency ... BigramFrequency ConsonantVowelProportion VisualComplexity
748 ruin 3.600000 2.004321 ... 344.750000 0.500000 52.606471
629 special 2.550000 3.526598 ... 371.714286 0.571429 64.664890
506 bore 3.550000 2.068186 ... 786.250000 0.500000 68.910505
459 prospect 2.800000 2.732394 ... 554.000000 0.750000 67.446434
804 craze 3.200000 1.662758 ... 474.400000 0.600000 64.583106
183 sarcasm 3.150000 1.724276 ... 548.428571 0.714286 69.948661
417 transit 4.315789 2.152288 ... 721.571429 0.714286 55.318946
536 moral 2.350000 3.014521 ... 727.800000 0.600000 64.202329
892 worship 2.900000 2.336460 ... 418.714286 0.714286 68.605603
65 smoke 5.550000 2.933993 ... 208.400000 0.600000 76.196174
[10 rows x 8 columns]
Now we are ready to create the “model” RDM, which will encode the difference in length between the words shown during the experiment.
rdm = mne_rsa.compute_rdm(epochs.metadata.NumberOfLetters, metric="euclidean")
# Plot the RDM
fig = mne_rsa.plot_rdms(rdm, title="Word length RDM")
fig.set_size_inches(3, 3) # Make figure a little bigger to show axis properly
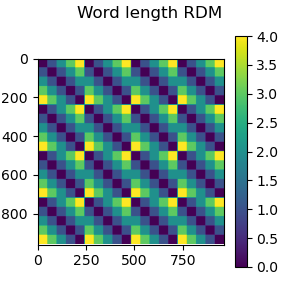
Total running time of the script: (0 minutes 1.498 seconds)